Predicting plant available phosphorus using infrared spectroscopy with consideration for future mobile sensing applications in precision farming (2020.0)
Pätzold S., Leenen M., Frizen P., Heggemann T., Wagner P., Rodionov A.
Precision Agriculture, 21 (4), 737-761
doi:10.1007/s11119-019-09693-3
Abstract
AbstractPhosphorus (P) fertilisation recommendations rely primarily on soil content of plant available P (Pavl) that vary spatially within farm fields. Spatially optimized P fertilisation for precision farming requires reliable, rapid and non-invasive Pavl determination. This laboratory study aimed to test and to compare visible-near infrared (Vis–NIR) and mid-infrared (MIR) spectroscopy for Pavl prediction with emphasis on future application in precision agriculture. After calibration with the conventional calcium acetate lactate (CAL) extraction method, limitations of Vis–NIRS and MIRS to predict Pavl were evaluated in loess topsoil samples from different fields at six localities. Overall calibration with 477 (Vis–NIRS) and 586 (MIRS) samples yielded satisfactory model performance (R2 0.70 and 0.72; RPD 1.8 and 1.9, respectively). Local Vis–NIRS models yielded better results with R2 up to 0.93 and RPD up to 3.8. For MIRS, results were comparable. However, an overall model to predict Pavl on independent test data partly failed. Sampling date, pre-crop harvest residues and fertilising regime affected model transferability. Varying transferability could partly be explained after deriving the cellulose absorption index from the Vis–NIR spectra. In 62 (Vis–NIRS) and 67% (MIRS) of all samples, prediction matched the correct Pavl content class. Rapid discrimination between high, optimal and low P classes could be carried out on many samples from single fields thus marking an improvement over the common practice. However, Pavl determination by means of IR spectroscopy is not yet satisfactory for determination of precision fertilizer dosage. For introduction into agricultural practice, a standardized sampling protocol is recommended to help achieve reliable spectroscopic Pavl prediction.
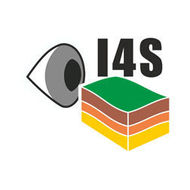